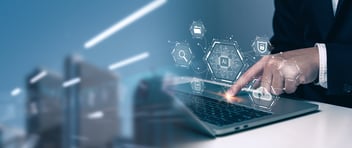
Generative AI is rapidly transforming the finance industry, offering unprecedented opportunities for efficiency, accuracy, and strategic insight. However, adopting these advanced technologies also presents significant challenges that must be addressed to fully realize their potential. This article explores the key hurdles finance professionals face when integrating generative AI into their operations and outlines practical steps for preparing for this technological revolution. By understanding and mitigating these challenges, finance leaders can harness the power of generative AI to drive innovation and maintain a competitive edge in an increasingly complex financial landscape.
High-Accuracy Calculations: Ensuring AI systems can perform calculations with the high level of accuracy required in finance. This involves developing robust algorithms and validation processes to verify the accuracy of AI-generated financial insights and recommendations. Companies must invest in high-quality data and advanced analytics tools to ensure the reliability of their AI systems.
Robust Validation Processes: Developing robust validation processes to verify AI-generated financial insights and recommendations. This can involve cross-referencing AI outputs with human analysis and historical data to ensure accuracy and reliability.
Securing Sensitive Financial Information: Protecting sensitive financial information when training AI models, especially if using cloud-based solutions. This requires implementing strong encryption and access controls to safeguard data from unauthorized access and cyber threats.
Implementing Strong Cybersecurity Measures: Implementing strong cybersecurity measures to prevent unauthorized access to AI systems handling financial data. This includes regular security audits, vulnerability assessments, and the use of advanced security technologies to protect against cyber threats.
Clear Guidelines for AI Use: Developing clear guidelines for the use of AI in financial decision-making processes. This includes defining the roles and responsibilities of AI systems and human users and establishing protocols for AI oversight and accountability.
Accountability Frameworks: Creating accountability frameworks for AI-assisted financial operations and reporting. This can involve setting up monitoring and reporting mechanisms to track AI performance and ensure compliance with regulatory requirements and ethical standards.
Mitigating AI Errors: Addressing the risk of AI generating plausible but incorrect financial information or advice. This requires implementing safeguards and human oversight to catch and correct AI errors and continuously improving AI algorithms to enhance their accuracy and reliability.
Human Oversight and Safeguards: Implementing safeguards and human oversight to catch and correct AI errors. This can involve regular audits and reviews of AI-generated outputs, as well as training finance professionals to identify and address potential AI issues.
Low-Risk Use Cases: Start with low-risk use cases like drafting routine financial reports or conducting preliminary data analysis. This allows companies to test and refine AI applications in a controlled environment, minimizing the risk of errors and disruptions.
Continuous Refinement and Expansion: Continuously refine and expand AI applications based on lessons learned from initial implementations. This involves gathering feedback from users, monitoring AI performance, and making necessary adjustments to improve accuracy and effectiveness.
Assessing Current Team Skills: Assess current team skills and identify gaps in AI knowledge. This helps companies understand their training needs and develop targeted training programs to build AI expertise within their finance teams.
Comprehensive Training Programs: Provide comprehensive training programs on AI applications in finance, focusing on both technical skills and strategic thinking. This ensures that finance professionals have the knowledge and skills needed to effectively use AI tools and technologies.
Dedicated AI Team: Consider creating a dedicated AI team within the finance department. This team can focus on developing and implementing AI solutions, ensuring that AI initiatives align with business goals and deliver tangible value.
Investing in AI Tools and Platforms: Invest in AI tools and platforms specifically designed for financial applications. This includes selecting the right technologies and vendors, as well as ensuring that AI systems integrate seamlessly with existing financial software and databases.
Seamless Integration with Existing Systems: Work with IT to ensure AI systems integrate seamlessly with existing financial software and databases. This involves developing joint strategies for data management, security, and AI infrastructure to support AI initiatives.
Joint Strategies for Data Management and Security: Develop joint strategies for data management, security, and AI infrastructure. This helps ensure that AI systems are robust, secure, and capable of handling the complex data requirements of financial applications.
Educating C-Suite Executives: Educate other C-suite executives on the potential of AI in finance. This involves showcasing successful AI implementations and highlighting the benefits of AI for financial operations and decision-making.
Advocating for AI Investments: Advocate for AI investments and showcase successful implementations to build organizational support. This helps secure the necessary resources and buy-in for AI initiatives, ensuring their success and sustainability.
Pacific Data Integrators Offers Unique Data Solutions Leveraging AI/ML, Large Language Models (Open AI: GPT-4, Meta: Llama2, Databricks: Dolly), Cloud, Data Management and Analytics Technologies, Helping Leading Organizations Solve Their Critical Business Challenges, Drive Data Driven Insights, Improve Decision-Making, and Achieve Business Objectives.