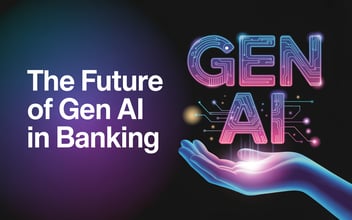
The Need for Smarter Decision-Making in Banking
Banks today face a storm of complexity: economic uncertainty, tightening regulations, rising customer expectations, and fierce competition from fintech disruptors. Traditional models where analysts pore over dashboards and executives interpret static charts often result in delayed or fragmented decision-making. (FintechMagazine)
Generative AI, particularly through large language models (LLMs), is rewriting this paradigm. These models don’t just crunch numbers; they explain, contextualize, and translate data into natural language insights. This enables leaders across functions from marketing to risk to compliance to act swiftly, without wading through layers of technical detail.
Imagine a scenario where a regional manager asks, “What’s the biggest risk to our mortgage portfolio this quarter?” Instead of waiting days for an analyst’s report, a generative AI assistant can instantly synthesize market data, customer trends, and regulatory changes, providing a clear, actionable summary within seconds.
One of the most stubborn pain points in banking is the fragmentation of data. Customer profiles, transaction histories, compliance records, and market intelligence often live in separate, disconnected systems. Integrating this data manually is time-consuming and prone to error.
Generative AI offers a powerful solution. By interfacing with multiple systems, it can aggregate, synthesize, and contextualize diverse data streams into a unified, coherent view. For example, a bank’s AI assistant can generate an executive briefing that combines customer churn trends, regional market developments, and internal sales performance. This dramatically reduces the manual effort of querying databases, pulling reports, and stitching together disparate insights.
Furthermore, leveraging retrieval-augmented generation (RAG) frameworks, banks can draw on both structured data (like transaction logs) and unstructured data (like regulatory filings or customer emails). This holistic, 360-degree perspective enables decision-makers to operate with greater confidence and precision.
Scenario Simulation and Strategic Forecasting
Traditional forecasting tools often rely on static models and historical assumptions, which can fall short in volatile environments. Generative AI introduces dynamic scenario simulation: the ability to model multiple futures based on evolving inputs.
Consider a retail bank debating an interest rate hike. With generative AI, leaders can simulate how such a move would affect customer retention, loan defaults, and net interest margins. The AI doesn’t just spit out raw numbers; it generates narrative scenarios, complete with projections, risks, and recommended actions. (HarvardBusinessReview)
This capability also transforms stress-testing. Instead of applying generic regulatory scenarios, banks can create AI-generated stress tests tailored to their specific portfolios, market exposures, and operational risks. This bespoke approach enables sharper risk management and better preparation for financial shocks or systemic disruptions.
Generative AI is not reserved solely for the C-suite. With fine-tuned, role-specific models, banks can democratize decision intelligence across all levels.
A relationship manager preparing for a high-value client meeting can receive AI-curated talking points, product recommendations, and cross-sell opportunities, all tailored to the client’s history and predicted needs.
A compliance officer can prompt the AI to summarize new regulatory mandates, flag potential compliance gaps, and suggest remedial actions.
A marketing analyst can generate fresh campaign ideas aligned with shifting customer behavior, as detected from transaction patterns and social sentiment.
This wide distribution of tailored insights enhances productivity, sharpens local decision-making, and fosters a culture of agility and responsiveness.
Risk-Aware Decisioning with Transparent Rationale
A common criticism of AI systems is their lack of transparency, the so-called "black box" problem. In highly regulated industries like banking, opaque AI recommendations are simply unacceptable.
Generative AI, when designed with explainability in mind, offers a solution. Not only can these models provide recommendations, but they can also deliver the rationale behind each suggestion. For instance, an AI might recommend adjusting lending policies by stating, “Due to rising delinquency rates among 18–24-year-olds and a projected increase in Q3 unemployment, reducing credit exposure in this demographic is advisable.”
This embedded reasoning fosters trust, supports regulatory compliance, and ensures that decisions are both defensible and auditable. (Legal transparency in AI finance: facing the accountability dilemma in digital decision-making | Reuters)
Compliance and Governance Considerations
Deploying generative AI in banking requires a rigorous approach to governance. Banks must put in place safeguards to manage:
Data provenance: Ensuring the AI only accesses authorized, anonymized datasets to protect customer privacy.
Bias mitigation: Regularly auditing outputs to detect and correct biases, particularly in sensitive areas like credit scoring, hiring, or customer segmentation.
Audit trails: Maintaining detailed logs of AI prompts, responses, and downstream decisions to meet regulatory and ethical standards. (IBM)
Notably, regulators around the world, from the EU AI Act to U.S. regulatory agencies, are intensifying scrutiny over how financial institutions deploy AI. Banks that proactively align with emerging compliance frameworks will not only avoid penalties but also build reputational trust.
Getting Started: A Practical Path Forward
For banks ready to harness generative AI for strategic decision-making, a clear, phased approach is essential:
Conclusion
Generative AI is more than just the latest tech trend; it is a strategic imperative for banks aiming to thrive in an unpredictable world. By unifying fragmented data, simulating future scenarios, and delivering actionable, transparent insights, generative AI transforms decision-making from a reactive process into a proactive, foresight-driven strategy.
Banks that seize this moment are not just optimizing operations; they are redefining leadership. They are building organizations that think faster, plan smarter, and act with confidence. In a landscape where agility, intelligence, and speed determine success, generative AI offers the ultimate decision advantage.
The time to act is now. Banks that pilot, learn, and scale generative AI capabilities today will position themselves as the innovators and market leaders of tomorrow, turning insight not just into action, but into lasting competitive edge.
You can book a consultation today by visiting us at PDI.
Pacific Data Integrators Offers Unique Data Solutions Leveraging AI/ML, Large Language Models (Open AI: GPT-4, Meta: Llama2, Databricks: Dolly), Cloud, Data Management and Analytics Technologies, Helping Leading Organizations Solve Their Critical Business Challenges, Drive Data Driven Insights, Improve Decision-Making, and Achieve Business Objectives.