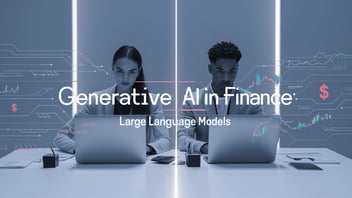
While the potential of AI and generative AI in finance is vast, its real-world applications and challenges are equally complex. This article delves into the cutting-edge use cases of generative AI that are reshaping financial institutions today. From automating compliance processes to revolutionizing loan approvals, we explore how banks and financial services firms are leveraging this technology to gain competitive advantages. We'll examine notable case studies that showcase successful implementations, and confront the hurdles – ethical, technical, and regulatory – that the industry must overcome. As generative AI continues to evolve, understanding these advanced applications and challenges is crucial for anyone looking to navigate the future of finance.
Automating compliance processes. AI systems can continuously monitor transactions and flag potential compliance issues, reducing the risk of regulatory violations.
Analyzing regulatory texts and legislative frameworks. AI can quickly process and interpret new regulations, helping institutions adapt their practices more efficiently.
Identifying potential compliance issues proactively. By analyzing patterns in historical data, AI can predict and highlight areas where compliance risks may emerge.
Automated loan application evaluation. AI can process applications much faster than human reviewers, considering a wider range of factors in decision-making.
Expedited approval processes. By automating routine checks and assessments, AI can significantly reduce the time from application to approval.
Enhanced creditworthiness assessments using diverse data sources. AI can analyze non-traditional data like social media activity or educational background to provide a more comprehensive view of an applicant's creditworthiness.
Analyze customer data. The bank uses AI to process vast amounts of customer transaction data and financial history.
Predict financial needs and preferences. Based on this analysis, the AI system anticipates customers' future financial requirements.
Offer personalized financial advice and product recommendations. The system generates tailored suggestions to help customers achieve their financial goals.
Analyze large datasets. Aiden processes market data, news, and other relevant information at a scale impossible for human analysts.
Optimize investment strategies. The platform uses this analysis to refine and improve investment approaches continuously.
Provide enhanced investment recommendations. Aiden generates insights that help RBC offer more informed and timely advice to its clients.
Enhanced risk management practices. AI models help the bank identify and mitigate potential risks more effectively.
More accurate credit scoring models. By incorporating a wider range of data points, the bank's AI-driven credit scoring provides a more nuanced assessment of creditworthiness.
Improved customer experiences. AI-powered systems enable the bank to offer more personalized services and faster response times.
Download Whitepaper: Leveraging AI Algorithms for Enhanced Finance Operations |
![]() |
Do not perpetuate biased decision-making. This requires careful monitoring and adjustment of AI models to prevent unfair treatment of certain customer groups.
Protect customer privacy. As AI systems process vast amounts of personal data, robust safeguards must be in place to prevent breaches or misuse.
Operate with transparency and accountability. Financial institutions need to be able to explain how AI-driven decisions are made, especially in areas like loan approvals or investment recommendations.
Sufficient computing power. Processing the vast amounts of data needed for effective AI models demands significant computational resources.
Adequate data storage capabilities. Financial institutions need robust infrastructure to securely store and manage the large datasets used in AI applications.
Skilled personnel to develop and maintain AI models. There's a growing demand for data scientists and AI specialists who understand both the technology and the financial sector.
Navigate emerging regulations. Governments and regulatory bodies are developing new rules for AI use in finance, which institutions must stay ahead of.
Adhere to guidelines for responsible AI use. This includes ensuring fairness, transparency, and accountability in AI-driven processes.
Stay informed about policy developments. The regulatory landscape for AI in finance is rapidly evolving, requiring constant vigilance and adaptation.
Pacific Data Integrators Offers Unique Data Solutions Leveraging AI/ML, Large Language Models (Open AI: GPT-4, Meta: Llama2, Databricks: Dolly), Cloud, Data Management and Analytics Technologies, Helping Leading Organizations Solve Their Critical Business Challenges, Drive Data Driven Insights, Improve Decision-Making, and Achieve Business Objectives.