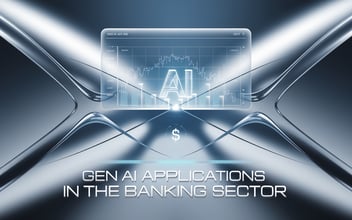
While Generative AI (GenAI) offers numerous benefits to the financial sector, its implementation comes with a set of challenges and risks that financial institutions must carefully navigate. Understanding and addressing these issues is crucial for successful adoption and responsible use of GenAI in finance.
Large Energy Requirement: GenAI systems, particularly those based on large language models, often require significant computational power. This high energy consumption can lead to increased operational costs and strain on IT resources. For instance, training a single large language model can consume as much energy as several households use in a year. Financial institutions must consider the environmental impact and cost implications of running these energy-intensive systems.
Data Quality Issues: The adage "garbage in, garbage out" is particularly relevant for GenAI in finance. Poor input data can lead to inaccurate or unreliable outputs, potentially resulting in significant miscommunications or falsified results. For example, if a GenAI system is trained on inaccurate or outdated financial data, it could generate misleading financial reports or flawed investment recommendations. Ensuring data quality is critical, but it's also a significant challenge given the vast amounts of data these systems process.
Cybersecurity Threats: GenAI systems in finance are vulnerable to various cybersecurity risks. These systems often rely on large amounts of sensitive financial data, making them attractive targets for hackers and malicious actors. A breach could lead to unauthorized access to sensitive financial information, financial fraud, or market manipulation. For instance, a compromised GenAI system could be manipulated to generate false financial reports or misleading market analyses, potentially causing significant financial losses and reputational damage.
Governance and Regulatory Compliance: The use of GenAI in finance raises complex governance and regulatory compliance challenges. Financial institutions need to ensure that their GenAI systems comply with industry regulations and guidelines, including those related to transparency, explain ability, and fairness in decision-making processes. For example, if a GenAI system is used in credit scoring, institutions must be able to explain how the system arrives at its decisions to comply with fair lending regulations. Adhering to these requirements while leveraging the full potential of GenAI can be a delicate balancing act.
Data Privacy and Security: GenAI systems in finance often process vast amounts of sensitive personal and financial data. Ensuring the privacy and security of this information is paramount. Financial institutions must implement robust data protection measures, including encryption, access controls, and data anonymization techniques. They must also comply with data protection regulations like GDPR or CCPA, which can be challenging given the complexity and scale of GenAI systems.
Bias and Fairness: GenAI systems can inadvertently perpetuate or amplify biases present in their training data. In finance, this could lead to unfair treatment of certain customer groups or skewed investment decisions. For instance, a GenAI system trained on historical lending data might discriminate against certain demographics if those biases were present in the historical data. Detecting and mitigating these biases is a significant challenge that requires ongoing monitoring and adjustment.
Explainability and Transparency: Many GenAI systems, particularly those based on deep learning, operate as "black boxes," making it difficult to understand how they arrive at their conclusions. This lack of explainability can be problematic in finance, where decisions often need to be justified to regulators, shareholders, or customers. For example, if a GenAI system recommends denying a loan application, the bank needs to be able to explain the reasoning behind this decision.
Download Whitepaper: Leveraging AI Algorithms for Enhanced Finance Operations |
![]() |
Pacific Data Integrators Offers Unique Data Solutions Leveraging AI/ML, Large Language Models (Open AI: GPT-4, Meta: Llama2, Databricks: Dolly), Cloud, Data Management and Analytics Technologies, Helping Leading Organizations Solve Their Critical Business Challenges, Drive Data Driven Insights, Improve Decision-Making, and Achieve Business Objectives.